Healthcare has significantly changed over the years, and technology is one of the reasons for these tremendous changes. Artificial Intelligence (AI), specifically, is emerging to be taking healthcare into the 21st century. For people involved in these industries, this isn’t a surprise, as technological innovations through the years have always influenced healthcare.
These technological innovations not only help in drug development, but they also facilitate accurate and rapid diagnosis of various diseases, treatment, research and development, tools, fraud detection, and a lot more. AI, and its various subsets like machine learning, deep learning, computer vision (of which semantic segmentation is one of the subcategories), and others, have pushed healthcare to a whole new level.
Overview Of Semantic Segmentation
As one of computer vision’s subcategories, semantic segmentation is an algorithm that makes it possible to train computers in mimicking the way a human brain process and interpret images. It involves classifying individual pixels found in images and putting a label on each of them. The label would then be linked to a certain class.
This would indicate that the pixels in the images are paired with the class they’re identified with. Semantic segmentation is therefore useful in grouping various objects with their own category. It's the method of designating a label to the pixels in an image.
An image of three Chihuahuas on a table, for example, would be processed by computer vision using semantic segmentation this way: first, the algorithm would label each Chihuahua as ‘dog.’ Those pixels found in the image of the dogs would then be identified and categorized further as ‘dog.’ The algorithm learns to do this by being fed millions of data images. The more that it's fed data, the more that it learns.
Semantic segmentation, working as an integral part of computer vision and under AI, can process petabytes of image data quickly and accurately. It’s a very useful tool, and it’s actually used in many industries besides healthcare.
Healthcare Applications Of Semantic Segmentation
The increase in computing power and the availability of data-enabled artificial intelligence and its subsets, like machine learning, deep learning, including computer vision and semantic segmentation, to develop further. Computer engineers were able to develop sophisticated algorithms due to the increased capabilities of computer hardware.
The availability of data for processing, on the other hand, helped artificial intelligence to ‘learn’ and be trained; as a result, big data and artificial intelligence developed a kind of synergistic relationship—both depending on one another to produce something greater. Here are a few results when AI, through computer vision with semantic segmentation, is used in healthcare:
1- Accurate Diagnoses
Semantic segmentation found one of its niches in diagnostic applications, which resulted in, among other things, lessening instances of invasive surgical procedures on patients that are done for diagnostic purposes. It’s now possible to detect anomalies on patients’ vital organs, such as the lungs, heart, liver, and others without resorting to surgery.
Software that features computer vision with semantic segmentation can be incorporated on MRI machines, where a patient’s organs can be viewed in 3D format and analyzed on a computer monitor. These 3D images are enough for the radiologist to accurately gauge the condition of the patient’s vital organs without the patient going under the knife. Semantic segmentation makes the process more accurate and can detect any abnormalities that a human eye might have missed.
2- Medical Imaging
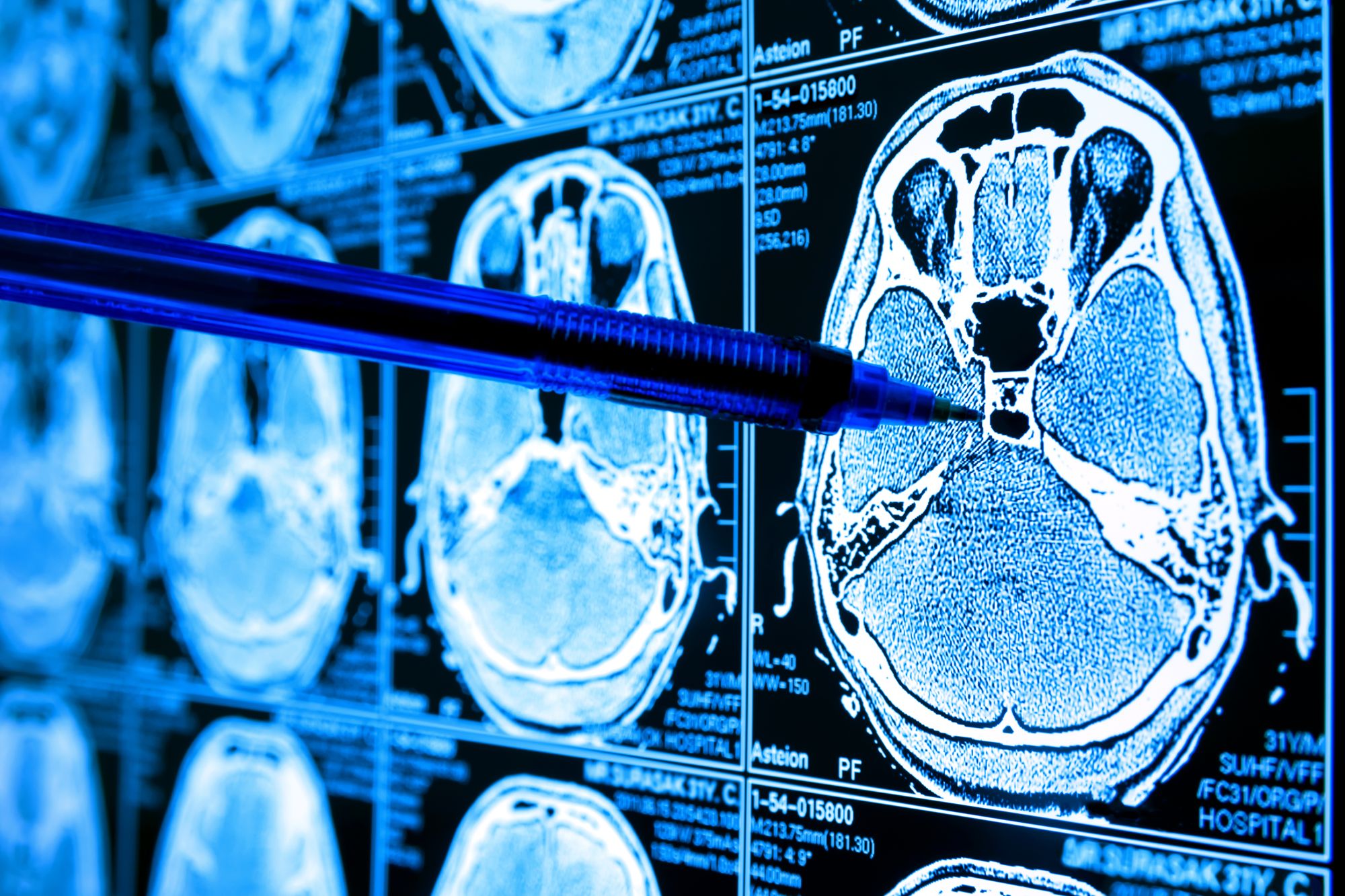
Medical imaging, which includes X-rays, 2D echoes, and others, isn’t exactly new to healthcare. But with the development of algorithms like semantic segmentation, medical professionals now have the tools to make a well-informed assessment of a patient’s condition. It’s now possible for 2D medical images to be rendered into 3D, enabling doctors to get a better picture of the patient’s health concerns.
Semantic segmentation also made it possible for computer vision to detect abnormalities such as tumors in X-rays. All it takes is for the radiologist to upload images of the X-ray scans into a software program, which would then mark the parts with tumors. The radiologist would then analyze the result and make the proper verdict.
3- Cancer Detection
There are forms of cancer that are not easy to detect. Skin cancer, for example, is notoriously difficult to catch in its early stages, as its symptoms are quite similar to garden-variety skin problems. But thanks to semantic segmentation and machine learning, detecting cancer in the early stages is now possible.
Scientists were able to develop apps that can pinpoint cancerous lesions on the epidermis (the skin’s outer layer), differentiating them from non-cancerous ones. With semantic segmentation, neural networks, machine learning, and other subcategories of computer vision, scientists have built a computer model (a program that simulates various possibilities that could happen) that has been trained in diagnosing skin cancer accurately.
They trained the computer model by ‘feeding’ it with a dataset of over a million skin cancer images. This is particularly helpful, as it can potentially save lives. In the U.S., about 5.4 million cases of skin cancer are diagnosed annually. The survival rate for this type of cancer, if detected early, is 97%.
However, the survival rate drops to around 14% if diagnosed in the later stages. With this application, skin cancer can be detected early, giving patients a higher chance of beating this disease. Other applications for identifying other types of cancer, such as breast and bone cancer, are also in the works.
4- Surgical Procedures
Computer models are also used to reduce the risks for patients who are undergoing surgical procedures. Applications like these help surgeons to prepare more thoroughly before doing invasive procedures, thereby reducing complications that might arise during surgery.
One application that semantic segmentation helped enable can estimate real-time blood loss, both during surgery and postoperative. This aids surgeons to accurately determine the amount of blood that the patient might need during and after surgery.
5- Research Applications
One of the AI applications that use semantic segmentation can track and identify a disease’s progression in certain patients. It can give crucial insights regarding the disease’s response, or lack of it, to certain medications. This could prove to be an important factor in decreasing the time and efforts that are spent when conducting clinical trials. In time, the app has the potential to be used to identify methods for preventing the development of certain diseases.
Final Thoughts
One of the greatest beneficiaries of the advancement of technology is in healthcare. As artificial intelligence continues to develop, more and more healthcare applications are created, ranging from fast and accurate diagnoses to assisting surgeons in performing surgery.
Semantic segmentation is a crucial part of computer vision, which is a subcategory of artificial intelligence. Without a workable semantic segmentation and its many variants, all these healthcare applications won’t be possible. It’s safe to say that the future of healthcare will be led by further advancements in artificial intelligence and its subsets.