Unlocking the Power of Artificial Intelligence in Healthcare: My Personal Journey
Table of Content
As a healthcare professional turned tech enthusiast, I've had the privilege of exploring the intersection of medicine and technology. My personal journey has been a fascinating one, filled with excitement and discovery.
In this article, I'll share my experiences with Large Language Models (LLMs) and Retrieval-Augmented Generation (RAG) systems, highlighting their potential to revolutionize healthcare.
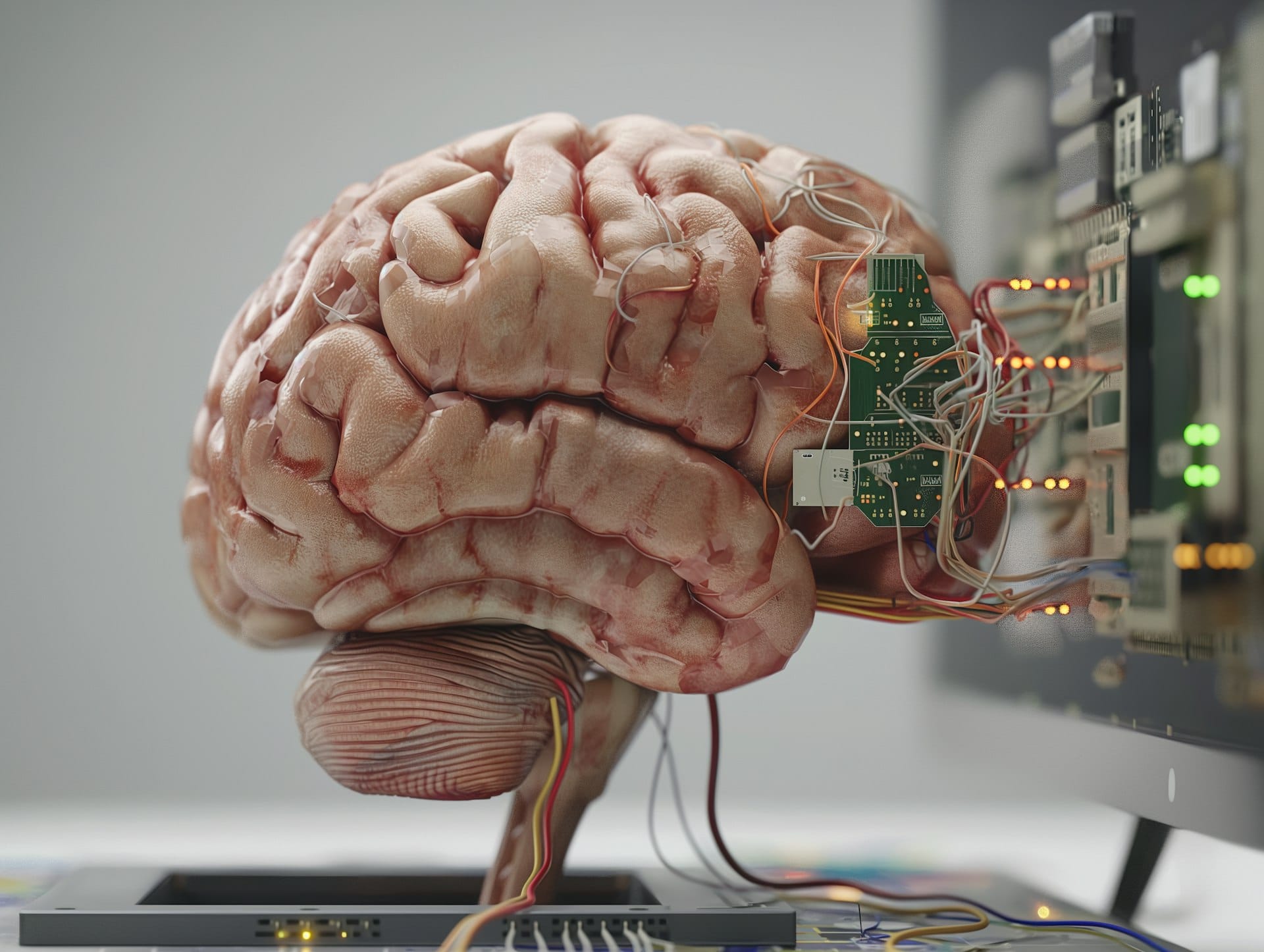
The Spark: A Medical Doctor's Perspective
As a doctor who is also involved in tech projects as a, I've always been drawn to the latest medical research and guidelines. However, staying up-to-date with the ever-changing landscape of healthcare can be overwhelming.
That's when I discovered LLMs and RAG systems, which promised to streamline data summarization, enhance diagnostic support, and improve clinical decision-making.
The Lowdown: What Are LLMs and RAGs?
LLMs (Large Language Models):
These AI models are like superheroes, capable of processing and generating human-like text. Trained on vast datasets, they can summarize information, provide contextual recommendations, and even understand complex language.
RAG (Retrieval-Augmented Generation):
This technology combines the reasoning power of LLMs with real-time knowledge retrieval from external databases or sources. Think of it as a dynamic duo, working together to provide accurate and unbiased information.
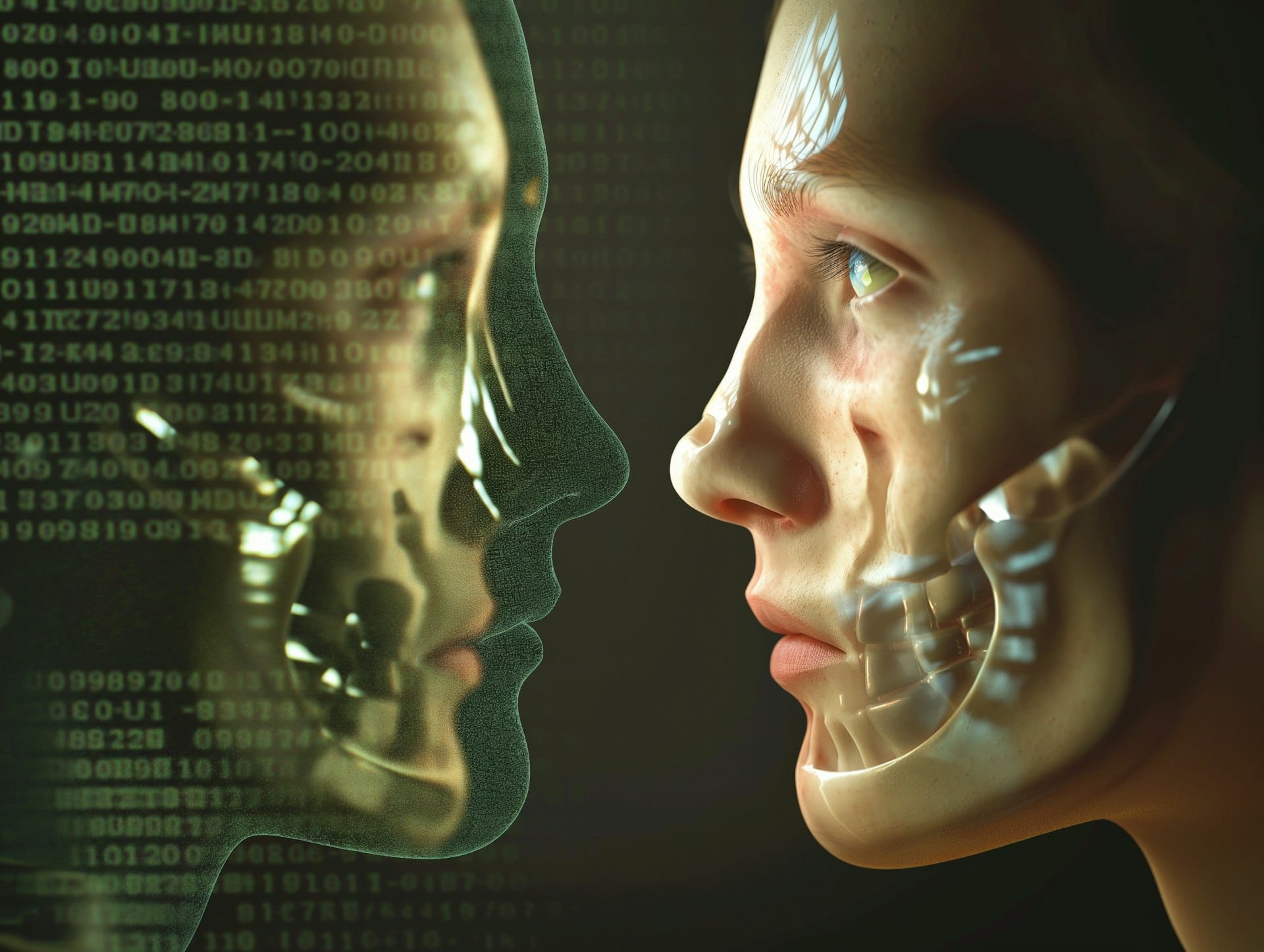
Challenges and Triumphs: Integrating LLMs and RAG into Medical Records
1- Information Overload:
Medical records are often cluttered with vast amounts of data, making it difficult to sift through and find relevant information.
2- Fragmented Systems:
Many hospitals and clinics use isolated systems that don't communicate effectively, leading to a lack of unified patient histories.
Benefits of integrating LLMs & RAG system with medical records.
However, with LLMs and RAG systems, we can overcome these challenges:
- Streamlined Data Summarization: Auto-generation of clinical notes and summaries saves time, allowing doctors to focus on patient care.
- Enhanced Diagnostic Support: RAG systems provide real-time access to institutional knowledge, enabling doctors to make informed decisions.
- Improved Clinical Decision-Making: LLMs can flag potential concerns, such as drug interactions or early signs of chronic conditions, ensuring timely interventions.
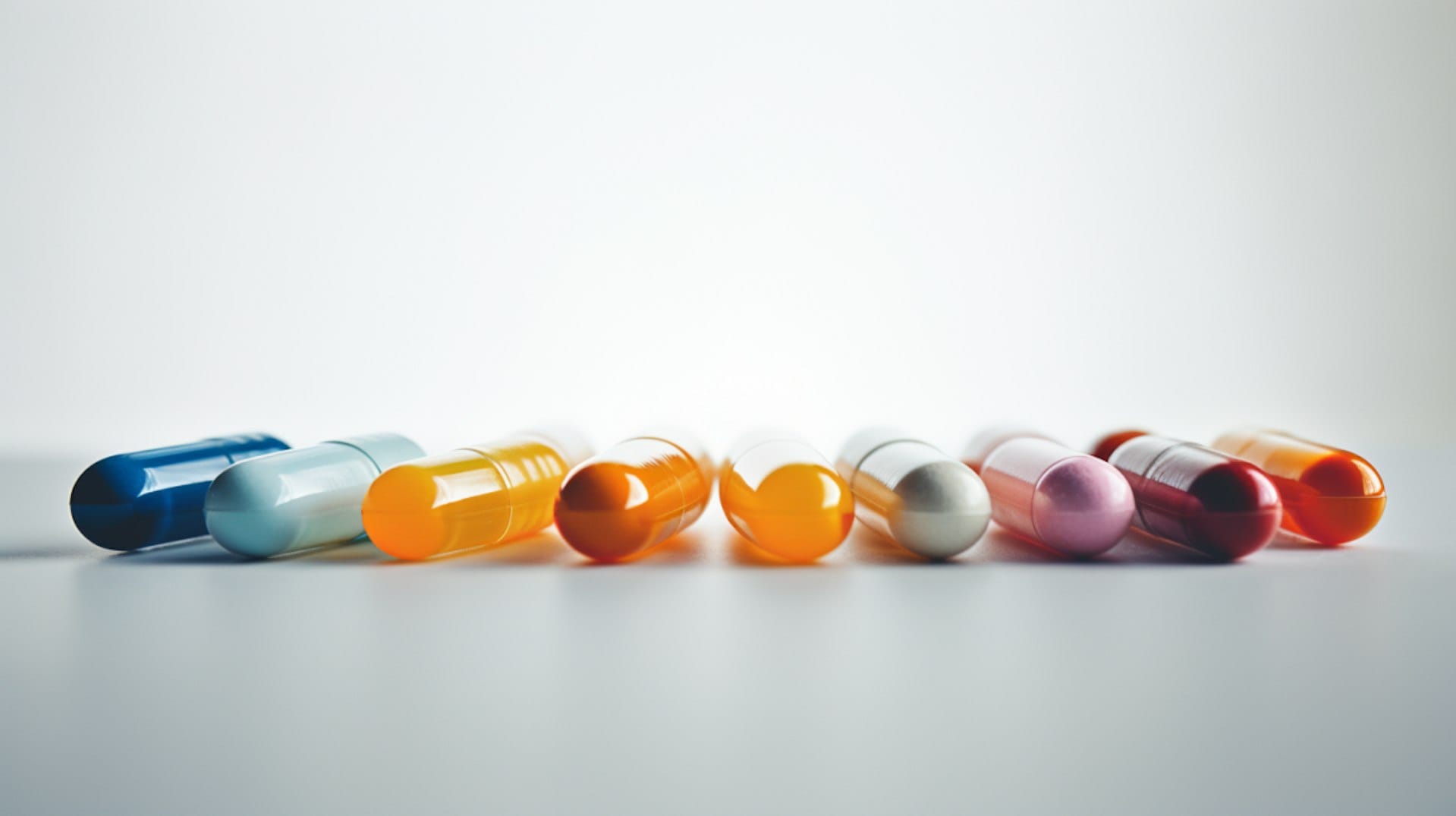
Benefits of Using LLMs and RAGs for Healthcare Providers and Organizations
1- For Doctors
- Faster Documentation: Auto-generation of clinical notes and summaries saves time.
- Reduced Cognitive Load: Decision support tools highlight critical patient data, enabling focus on care.
- Better Outcomes: Evidence-based recommendations improve diagnostic accuracy.
2- For Healthcare Organizations
- Increased Efficiency: Automating administrative tasks like coding or claim generation streamlines workflows.
- Scalability: RAG systems provide real-time access to institutional knowledge, even in large networks.
- Enhanced Patient Satisfaction: Improved communication fosters trust and adherence to treatment plans.
Success Stories: Real-World Applications
- GPT-4 (OpenAI): Used for summarization, natural language understanding, and conversational AI in healthcare apps.
- Meta’s LLaMA: An open-source LLM ideal for custom healthcare applications due to its flexibility.
- Google’s Med-PaLM: A healthcare-specific LLM that understands medical queries and provides answers based on scientific literature.
The Future of Healthcare: Embracing AI
As a community, let's work together to build solutions that make a difference. By leveraging tools like GPT-4, LangChain, and Haystack, we can revolutionize the way we care for patients.
The Key Takeaways
- Efficient Data Summarization: Auto-generation of clinical notes and summaries saves time.
- Enhanced Diagnostic Support: RAG systems provide real-time access to institutional knowledge.
- Improved Clinical Decision-Making: LLMs can flag potential concerns, ensuring timely interventions.
The Bottom Line
Integrating LLMs and RAGs with medical records represents a significant leap forward in how we approach patient care. From summarizing complex data to providing real-time decision support, these technologies empower doctors and healthcare organizations to deliver better, faster, and more personalized care.
As both a doctor and developer, I find this intersection of medicine and technology to be one of the most exciting areas to work in.
By leveraging tools like GPT-4, LangChain, and Haystack, we’re not just improving workflows but truly transforming the way we care for patients.
For those looking to adopt these technologies, start small—experiment with open-source tools, pilot programs, and specific use cases. The future of healthcare is here, and it’s powered by AI.
Open-source LLM an RAG tools!
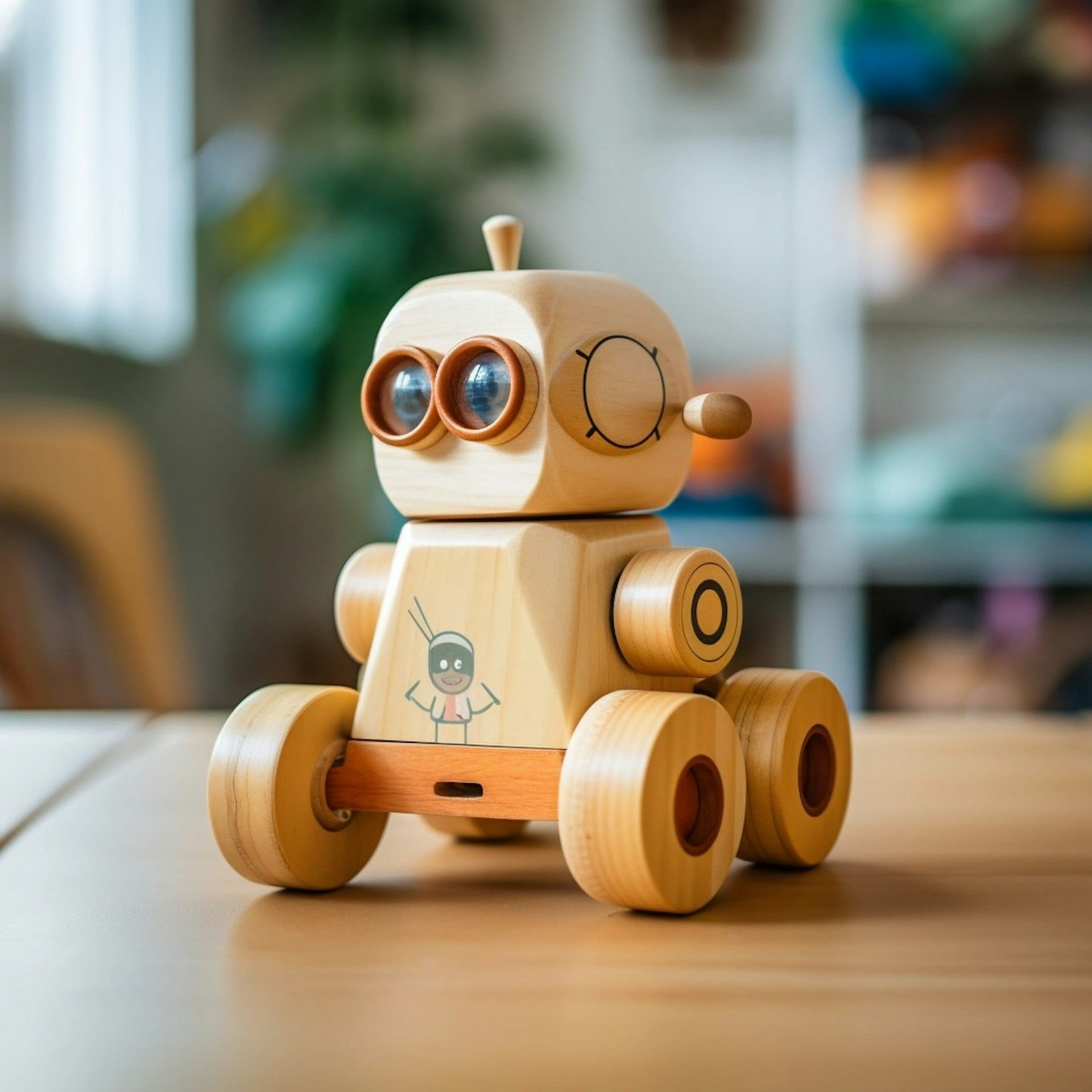
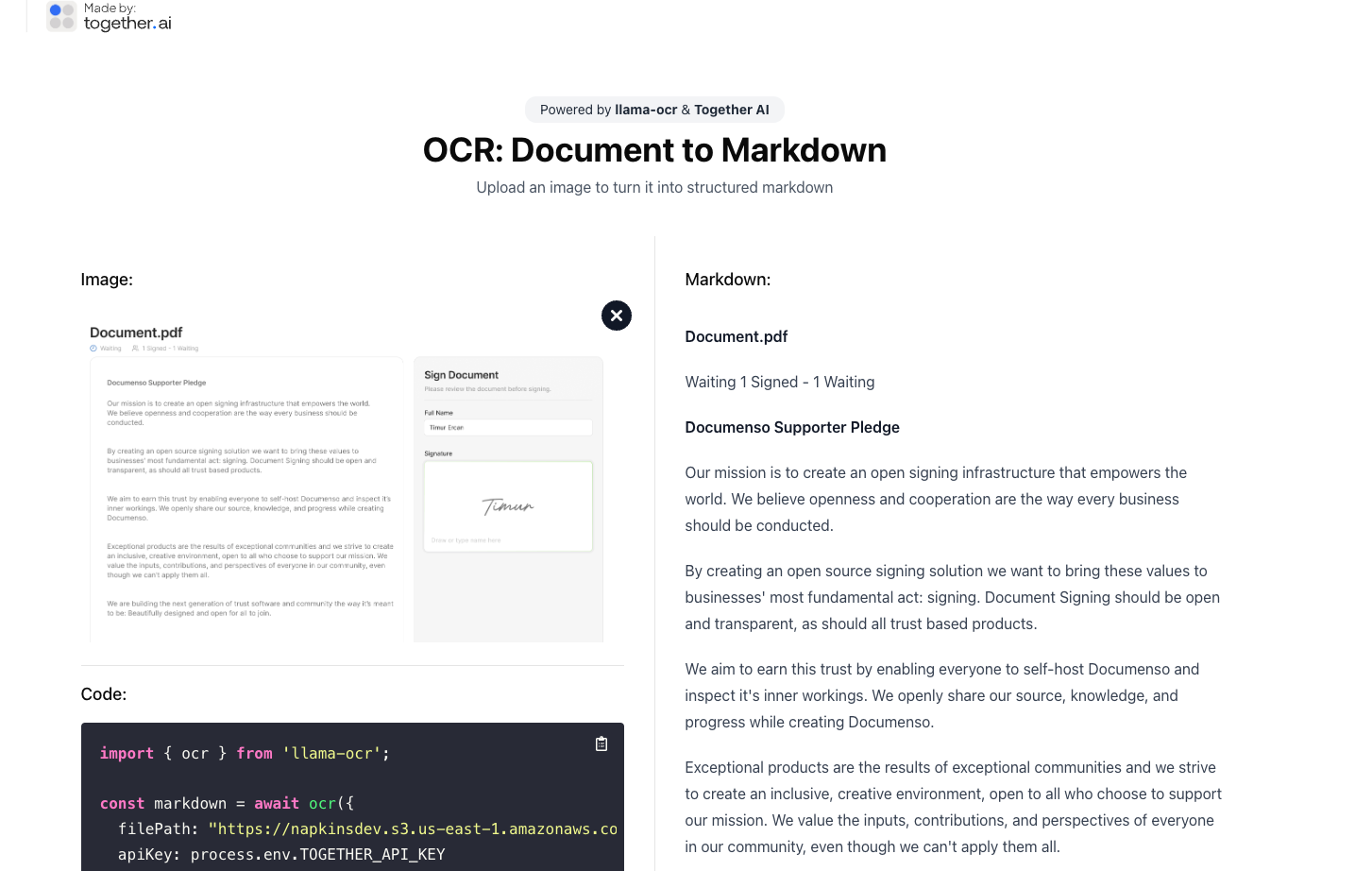
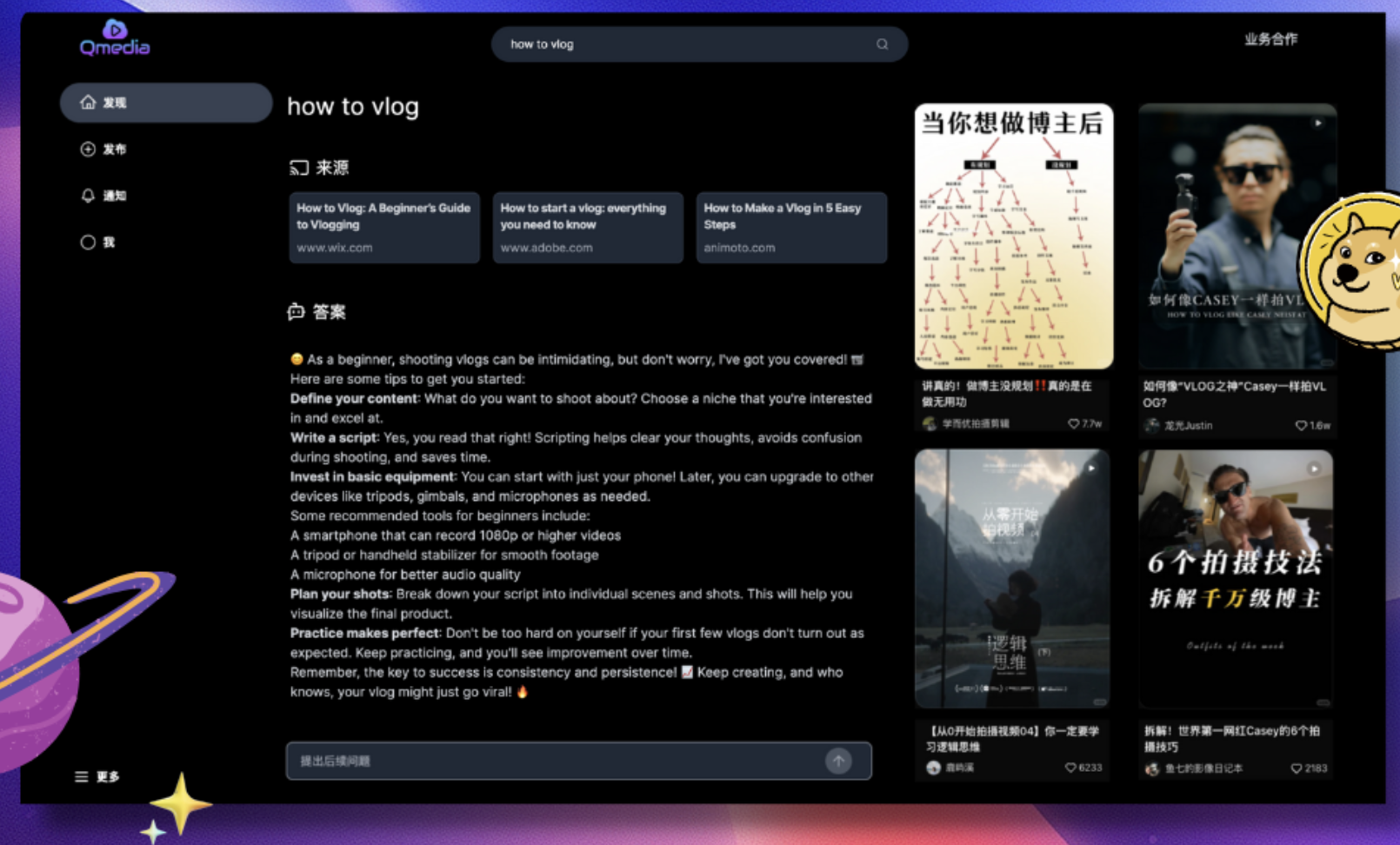
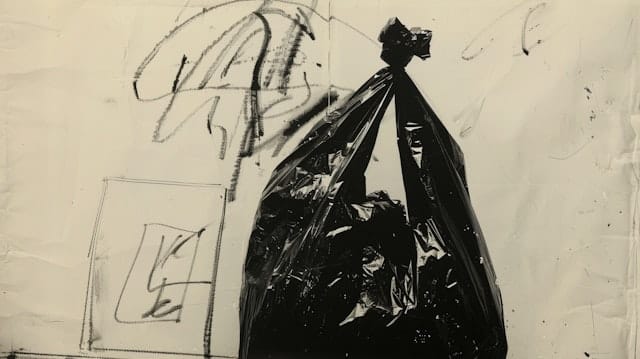
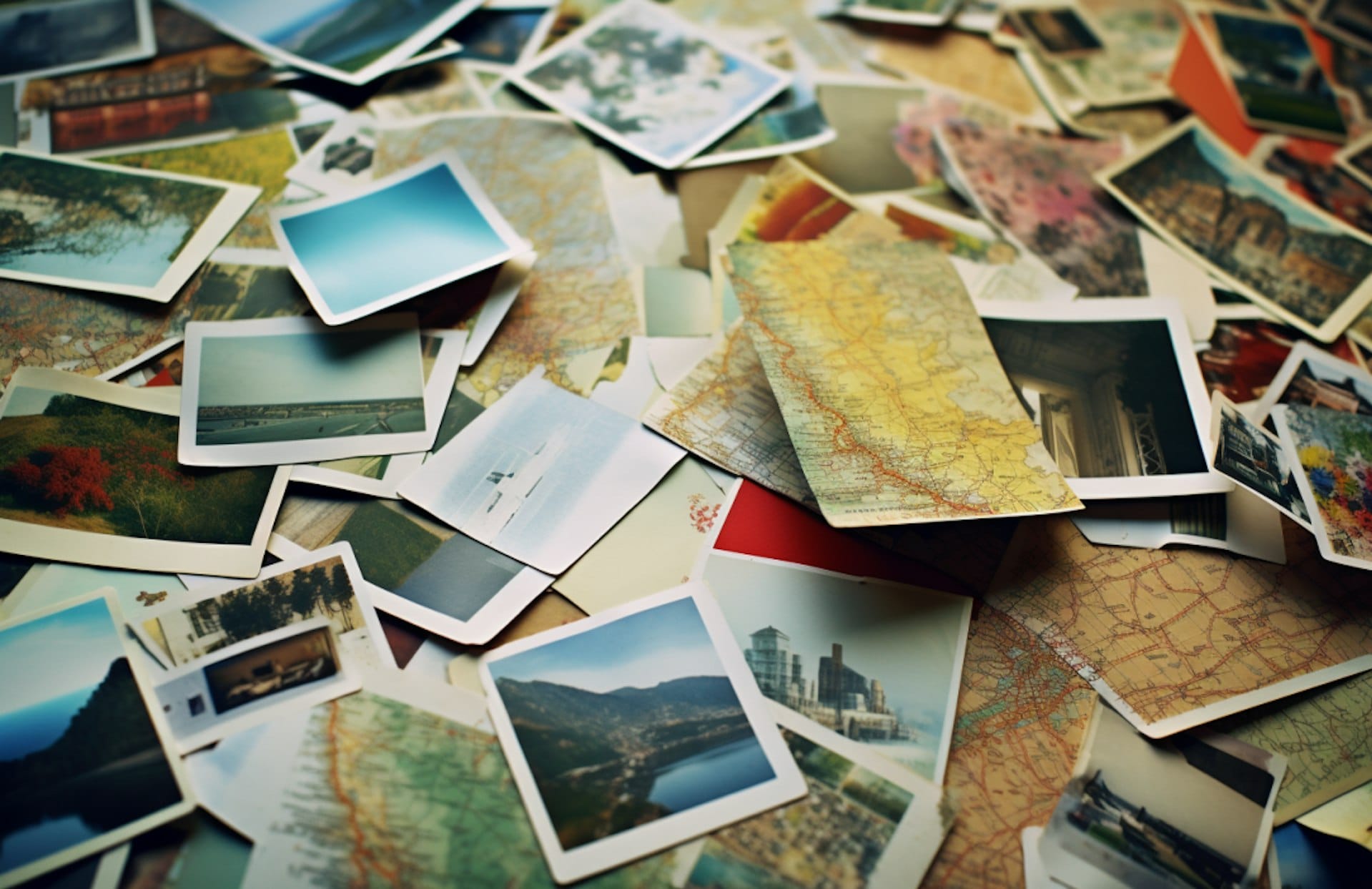
